What We Do
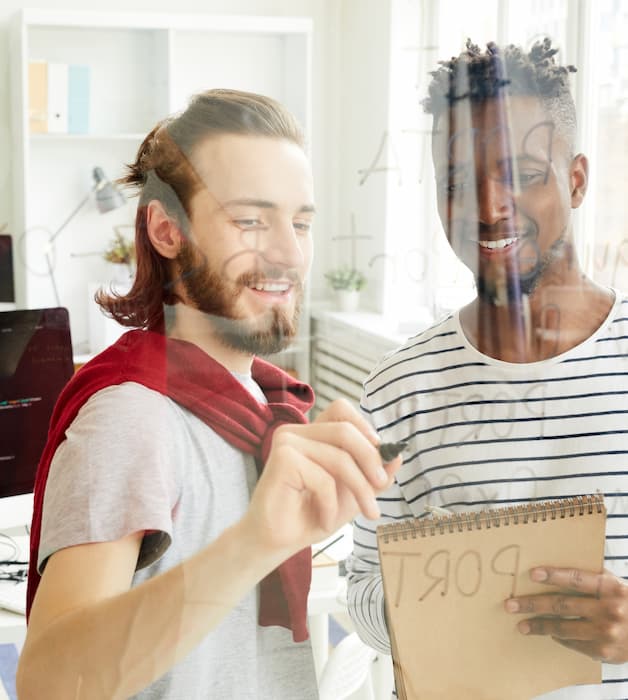
Who We Are
NobleProg is an international training and consultancy group, specializing in custom, live instructor-led training on the newest technologies.
弊社についてPopular Courses
コースカタログ
- 3Dモデリング
- Automotive
- DevOps
- DaDesktop
- Enterprise Resource Planning (ERP)
- IoT(Internet of Things, モノのインターネット)
- ISO Standards
- Linux
- MLOps
- Microsoft
- OMG
- Web開発
- Webサービス
- Webサーバー
- Windows OS
- ビッグデータ
- コンテナと仮想マシン(VM)
- データベース
- ブロックチェーン
- オペレーティングシステム(Operating System:OS)
- モバイルアプリ開発
- データ分析
- コンピューター・プログラミング
- クラウド・コンピューティング
- ソフトウェア工学
- ゲーム開発
- バーチャル・リアリティ(VR)
- アプリケーション性能管理(APM)
- グラフの計算
- サービス指向アーキテクチャ(SOA)
- ロボティック・プロセス・オートメーション(RPA)
- ロボット工学
- バージョン管理システム
- マイクロサービス
- アプリケーション・サーバー
- デスクトップパブリッシング(DTP)
- エンタープライズアーキテクチャ
- コンテンツ管理システム(CMS)
- ビジネスプロセス管理(BPM)
- データサイエンス
- ファイナンス
- カスタマー・リレーションシップ・マネジメント(CRM)
- ソフトスキル
- サイバー・セキュリティ
- モバイルネットワーク
- ネットワーキング
- コンピュータグラフィックス
- マーケティング
- データ管理
- プロジェクト管理
- ストリーミング
- コラボレーション
- グラフィック・デザイン
- インダストリアル・オートメーション
- バイオテクノロジー
- ビジネス
- ユーザー・エクスペリエンス(UX)デザイン
- 人工知能(AI)
- 分散システム
- 機械学習
- 拡張現実(AR)
- 検索
- 構成管理
- 統計
- 組み込みシステム
- 管理
- 設備資産管理(EAM)